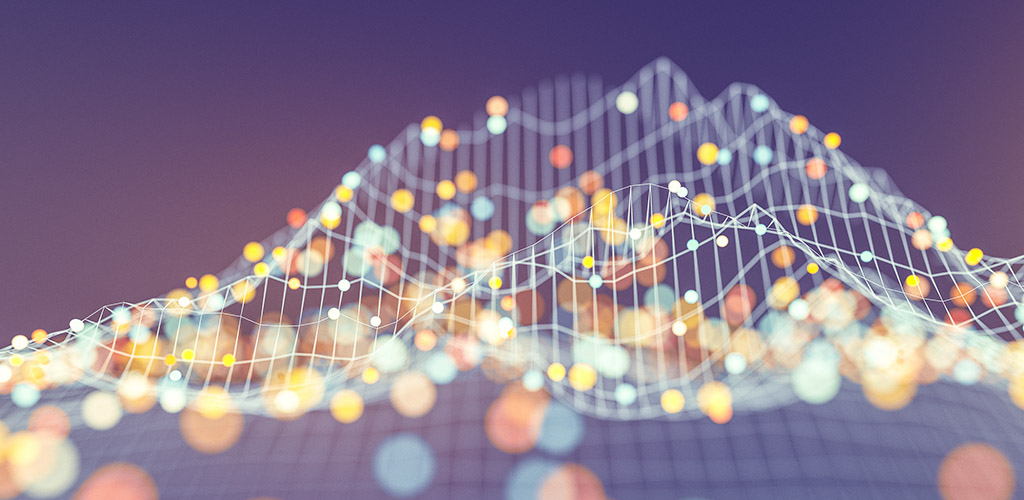
Nowadays, biomedical and social science researchers have access to data more than ever before. These data can provide extremely useful insights to describe, investigate and predict health and social outcomes with the consequent potential of improving the quality of our lives.
However, mishandling of data, or misinterpretation of results drawn from them, can lead to erroneous conclusions. As the public has gained an interest in public health research, especially since the start of the pandemic, it is paramount that data are treated correctly, and results are communicated effectively.
Indeed, there are many examples where misinterpretations occurred during this period. Papers published before 2020 on the effectiveness of masks in specific settings were inappropriately transposed to the setting of the COVID-19 pandemic with the scope of discouraging members of the public from wearing them. In 2021, statistics on those who tested positive for COVID-19 following a COVID-19 vaccination were also misinterpreted, leading to a substantial amount of people to believe that it was safer to avoid the vaccine.
The misunderstandings of these statistics had an impact on human behaviour, and as such stifled the effectiveness of public health policies which made attempts to constrain the spread of the disease. As a result, there is an increasing demand for statistical fluency to understand biases within data, interpretation of results and validity of subsequent conclusions.
This was not the only example of data failings during the COVID-19 pandemic. Due to the availability of data, many of the statistics published disproportionately over-represented white people, with limited generalisability to other groups. Thus, many marginalised groups grew disillusioned with COVID-19 reports, as these summary statistics did not describe their reality with COVID-19, an example of ecological fallacy on a wide scale. This impacted the trust of health advice coming from the government within these smaller communities.
This highlights the growing importance to utilise all available sources of data to gain better understanding of health dynamics in all societal groups. Ideally, information would be available from each individual in all communities, and over time, data would be available from diagnosis, to hospitalization, to recovery or death. These days, this type of information can be found from administrative sources, like general practitioner and school records, however, they may be incomplete and irregular over time. Therefore, these data sources need to be handled with care before extrapolating information.
Free training in longitudinal data science
Understanding the nature and the challenges of many types of data, especially those collected repeatedly over time, requires skills from different disciplines, including computer science, statistics, causal inference, and econometrics. As such, there is an increased need for health and social data scientists to undergo rigorous training to integrate such skills together.
RADIANCE is a UKRI-funded project that provides free online rigorous training in longitudinal data science to reach the broadest community of data scientists. It is led by Professor Bianca De Stavola and Professor Paola Zaninotto at University College London.
RADIANCE offers training in multiple forms. Short introductory videos called Appetisers are accessible via their website and YouTube channel in topics such as causal questions, information governance and trusted research environments. RADIANCE also offers short courses, called Modules, which take the form of taught lectures and live tutorials where the material covered by the lectures is reinforced with computer-based exercises using health data.
So far, RADIANCE has offered short courses on longitudinal data preparation and visualisation, addressing causal questions, using administrative data for research and regression models. Bookings are currently open for Multiple Imputation Of Missing Data.
For more information, or to sign up for free rigorous data in longitudinal data science, visit the RADIANCE website.