Introduction to Poisson regression models for count data
Presenter(s): Gabriele Durrant
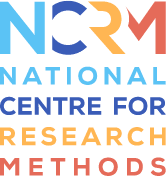
This resource introduces Poisson regression, a form of regression analysis which is used to model count data, such as number of event occurrences during a particular time period. For example, researchers may be interested in modelling the variation in the number of traffic accidents across a 12 months period or the number of stressful events experienced during the last 18 months prior to interview. Poisson regression assumes the response variable Y has a Poisson distribution, and assumes the logarithm of its expected value can be modelled by a linear combination of unknown parameters. Poisson regression models are generalized linear models with the logarithm as the link function. This web resource introduces the basic principles of Poisson regression. First, it discusses a very simple model (the equiprobable model), a model without a covariate. Goodness of fit statistics for Poisson regression are presented. It then extends the basic principles to a Poisson regression model with one covariate, and illustrates this with a Poisson time trend model.
Poisson regression models for count data
This first video of the series introduces the principles of Poisson regression modelling and presents a very simple model, the equiprobable model, which assumes that the number of events do not depend on time (e.g. weekdays or months), but are equally distributed across time. It uses an example to illustrate the principles.
Goodness of fit test statistics
This video presents goodness of fit statistics for Poisson regression. It introduces the Pearson and likelihood-ratio test statistics. Both are goodness-of-fit test statistics which compare two models, where the larger model is the saturated model (which fits the data perfectly and explains all of the variability) and the smaller model is the model of interest.
Diagnostic in poisson regression
This video extends the previous ideas to Poisson regression with a covariate. It discusses the example of a Poisson time trend model, where time (e.g. month) is the covariate. The interpretation of the coefficient is outlined and Goodness of fit statistics are applied. Possibilities for model interpretation are presented including the confidence interval for the regression coefficient (slope) and estimated change between time periods (e.g. between months).
About the author
Gabriele Durrant is Professor in Social Statistics and Survey Methodology in the Department of Social Statistics and Demography at the University of Southampton. She is currently leading research workpackage 1 of the ESRC National Centre for Research Methods on ?Data collection for data quality?, a 3-year ESRC project. She previously was the principle investigator of two 3- and 4-year ESRC-funded grants on nonresponse and paradata. She has research interests in the areas of paradata, interviewer effects, nonresponse in sample surveys, measurement error, and statistical modelling in the social sciences, in particular multilevel modelling. More recently she also developed an interest in using linked datasources, particularly in the area of energy use and environmental attitudes.
- Published on: 23 May 2016
- Event hosted by: National Centre for Research Methods
- Keywords: Poisson regression | count data | regression modelling | equiprobable model | goodness of fit | residual analysis |
- To cite this resource:
Gabriele Durrant. (2016). Introduction to Poisson regression models for count data. National Centre for Research Methods online learning resource. Available at https://www.ncrm.ac.uk/resources/online/all/?id=20429 [accessed: 22 November 2024]
⌃BACK TO TOP