Introduction to Latent Transition Analysis
Presenter(s): Oliver Perra
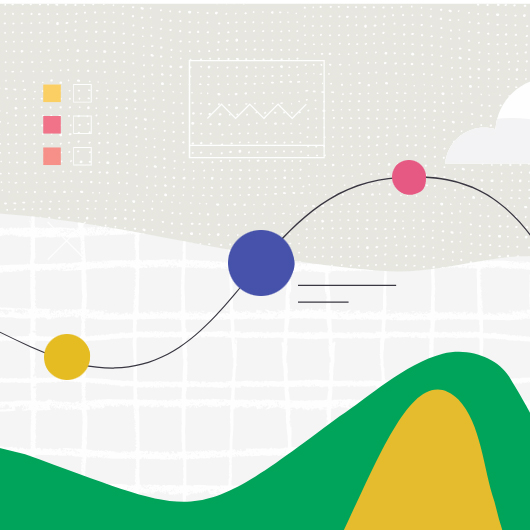
This resource illustrates key concepts and processes of Latent Transition Analysis (LTA), with examples from research and exercises using Mplus software (solutions to the exercises are also provided).
LTA is a Mixture Model approach applied to repeated measures and longitudinal data. Mixture models assume that a population is made up of sub-populations, a “mix” of individuals. These sub-populations share the same propensities to display some patterns of behaviour, and these propensities differ substantially from those of other sub-populations. It is therefore a person-centred approach: the focus is not on the relationships between variables, but rather on identifying groups of people that have similar characteristics or behave in similar ways.
LTA is basically an extension of latent class analysis to repeated measurements and longitudinal data. The first goal of LTA is to identify the best person-centred measurement models (latent class solutions) at each data measurement occasion. Namely, LTA allows to identify the sub-groups that at each time point explain inter-personal variation in the observed outcomes.
The second goal of LTA is to investigate structural relationships between classes at each time point. These analyses allow therefore to investigate patterns of continuity, individuals that remain in the same sub-groups across time points, as well as dis-continuity: how individuals transition from one sub-group to another one characterised by different behavioural propensities.
LTA is therefore an auto-regressive model of development: individuals’ status at each measurement occasion is a function of their status in the previous one. Change across measurement occasions is not usually modelled as a function of time. LTA is an ideal method to investigate stage-like and stadial models of development: (a) Its person-centred approach allows to identify novel behaviour patterns that emerge across measurement occasions; (b) It allows to formally test hypotheses regarding transitions that are considered unlikely (e.g. from being able to read fluently to struggling to read).
The purpose of LTA
The main assumptions of LTA rest on LCA: At each time point, persons in a sample belong to one latent class or another, i.e. latent classes are exhaustive and capture the whole of the sample. Another assumption is that each person at a given time belongs to one and only one of the latent classes: classes are mutually exclusive.
Another assumption is that of intragroup homogeneity: individuals within a class share the same propensity to behaviour patterns, and these propensities differ from those of individuals in other classes. The first goal of LTA is to enumerate the latent classes at each time point, characterise them in terms of how these underlying classes influence observed behaviours, and categorise individuals in these classes, based on the observed behaviour patterns.
The second goal of LTA is to investigate patterns of change across time by considering the probabilities of transitions from one latent class at one time point to other classes at a successive time, as well individuals’ probabilities to remain in the same classes. LTA also aims to investigate how covariates (e.g. socio-economic status) influence patterns of change or continuity across time.
Measurement Models
LTA provides a series of procedures to identify and characterise the optimal person-centred models to explain inter-personal variability at each data collection point. The emphasis on person-centred models allows to identify emerging sub-groups or classes that are characterised by novel behaviour patterns and propensities. LTA provides methods to formally test the fit of these measurement models across time and investigate measurement invariance of the underlying latent classes.
Structural Models
Methodological advancements have developed a novel approach to separate the LTA measurement modelling phase from the modelling of structural relationships. In fact, the Three-Step Approach conducts the measurement model estimation in two initial steps that allow estimation of respondents’ latent class affiliation and of the optimal LTA measurement parameters. The measurement parameters are fixed in the third step, whereby structural relationships between latent class variables at different time points, covariates of different types (e.g. other latent variables) and distal outcomes are modelled. This approach facilitates the specification of more complex models and their prompt estimation. Similar models have included investigation of moderation effects by covariates, models with multiple measurement occasions, and associative LTA where transition in person-centred latent variables across one process influence transitions in other processes.
___
Download the exercise files (Zip folder).
Exercises with solutions, datasets, and information about: LTA using Mplus, and stages of LTA.
___
Books and references:
Books
Hagenaars, J., & McCutcheon, A. (Eds.). (2002). Applied Latent Class Analysis. Cambridge: Cambridge University Press. doi:10.1017/CBO9780511499531
Collins, L. M., & Lanza, S. T. (2009). Latent class and latent transition analysis: With applications in the social, behavioral, and health sciences. New York: John Wiley & Sons. doi:10.1002/9780470567333
Introductions to LTA
Nylund-Gibson, K., Garber, A. C., Carter, D. B., Chan, M., Arch, D. A., Simon, O., ... & Lawrie, S. I. (2023). Ten frequently asked questions about latent transition analysis. Psychological Methods 28(2), 284-300. doi: 10.1037/met0000486.
Perra, O. (2020). Latent transition analysis. SAGE Research Methods: Foundations. doi: 10.4135/9781526421036878157
Ryoo, J. H., Wang, C., Swearer, S. M., Hull, M., & Shi, D. (2018). Longitudinal model building using latent transition analysis: An example using school bullying data. Frontiers in Psychology, 9, 675. doi:10.3389/fpsyg.2018.00675
References on approaches to include covariates and distal outcomes in LTA models
Asparouhov, T., & Muthén, B. (2014). Auxiliary variables in mixture modeling: Three-step approaches using Mplus. Structural Equation Modeling, 21, 329–341. doi:10.1080/10705511.2014.915181
Bray, B. C., Lanza, S. T., & Collins, L. M. (2010). Modeling relations among discrete developmental processes: A general approach to associative latent transition analysis. Structural Equation Modeling, 17, 541–569. doi:10.1080/10705511.2010.510043
Chung, H, Park, Y., & Lanza, S. T. (2005). Latent transition analysis with covariates: pubertal timing and substance use behaviours in adolescent females. Statistics in Medicine, 24, 2895–2910. doi:10.1002/sim.2148
Clark, S. L., & Muthén, B. (2009). Relating latent class analysis results to variables not included in the analysis. Retrieved from https://www.statmodel.com/download/relatinglca.pdf
Nylund-Gibson, K., Grimm, R., Quirk, M., & Furlong, M. (2014). A latent transition mixture model using the three-step specification. Structural Equation Modeling, 21, 329–341. doi:10.1080/10705511.2014.915181
Muthén, B., & Asparouhov, T. (2011). LTA in Mplus: Transition probabilities influenced by covariates. Mplus Web Notes, 13, 1-30. Retrieved from http://www.statmodel.com/examples/LTAwebnote.pdf
Vermunt, J. K. (2010). Latent class modeling with covariates: Two improved three-step approaches. Political Analysis, 18, 450–469. doi:10.1093/pan/mpq025
Other Advances in LTA
Muthén, B., & Asparouhov, T. (2022). Latent transition analysis with random intercepts (RI-LTA). Psychological Methods, 27(1), 1-16. doi:10.1037/met0000370
Associative LTA
Bray, B. C., Lanza, S. T., & Collins, L. M. (2010). Modeling Relations among Discrete Developmental Processes: A General Approach to Associative Latent Transition Analysis. Structural Equation Modeling-A Multidisciplinary Journal, 17(4), 541–569. https://doi.org/10.1080/10705511.2010.510043
Flaherty, B. P. (2008). Examining contingent discrete change over time with associative latent transition analysis. In G. R. Hancock & K. M. Samuelsen (Eds.), Advances In Latent Variable Mixture Models (pp. 77–103).
Flaherty, B. P. (2008). Testing the degree of cross-sectional and longitudinal dependence between two discrete dynamic processes. Developmental Psychology, 44(2), 468–480. https://doi.org/10.1037/0012-1649.44.2.468
Examples of Research in Substance Use
Wiginton, J. M., Booth, R., Eaton, L. A. A., Smith, L. R. R., da Silva, C. E., Patterson, T. L. L., & Pitpitan, E. V. V. (2023). Injection Drug Use and Sexual Risk Behaviors Among People who Inject Drugs in Ukraine: A Random-Intercept Latent Transition Analysis. Aids And Behavior. https://doi.org/10.1007/s10461-023-04024-0
Bray, B. C., Berglund, P. A., Evans-Polce, R. J., & Patrick, M. E. (2021). A Latent Transition Analysis of Self-Reported Reasons for Marijuana Use During Young Adulthood. Evaluation & The Health Professions, 44(1, Si), 9–24. https://doi.org/10.1177/0163278720984514
Bray, B. C., Lee, G. P., Liu, W., Storr, C. L., Ialongo, N. S., & Martins, S. S. (2014). Transitions in Gambling Participation During Late Adolescence and Young Adulthood. Journal Of Adolescent Health, 55(2), 188–194. https://doi.org/10.1016/j.jadohealth.2014.02.001
Cosden, M., Larsen, J. L., Donahue, M. T., & Nylund-Gibson, K. (2015). Trauma Symptoms for Men and Women in Substance Abuse Treatment: A Latent Transition Analysis. Journal Of Substance Abuse Treatment, 50, 18–25. https://doi.org/10.1016/j.jsat.2014.09.004
Crum, R. M., Green, K. M., Amin-Esmaeili, M., Susukida, R., Mojtabai, R., Storr, C. L., … Reboussin, B. A. (2022). The role of mood disorders in the progression of and recovery from alcohol and drug use problems: A latent transition analysis. Drug And Alcohol Dependence, 238. doi: 10.1016/j.drugalcdep.2022.109566
Dennermalm, N., Karlsson, P., & Ekendahl, M. (2023). Stability and change in substance use among Swedish adolescents: a latent transition analysis. Substance Use & Misuse, 58(7), 947-955. doi: 10.1080/10826084.2023.2198599
Hultgren, B. A., Turrisi, R., Cleveland, M. J., Mallett, K. A., Reavy, R., Larimer, M. E., … Hospital, M. M. (2019). Transitions in drinking behaviors across the college years: A latent transition analysis. Addictive Behaviors, 92, 108–114. https://doi.org/10.1016/j.addbeh.2018.12.021
Examples of Research in Cognition
Huang, F., Zhang, M., & Wang, S. (2019). Changes in cognitive function among older adults: A latent profile transition analysis. Archives Of Gerontology And Geriatrics, 80, 12–19. https://doi.org/10.1016/j.archger.2018.09.006
Swanson, H. L., Arizmendi, G. D., & Li, J.-T. (2021). The Stability of Learning Disabilities Among Emergent Bilingual Children: A Latent Transition Analysis. Journal Of Educational Psychology, 113(6), 1244–1268. https://doi.org/10.1037/edu0000645
Zammit, A. R., Bennett, D. A., Hall, C. B., Lipton, R. B., Katz, M. J., & Muniz-Terrera, G. (2020). A Latent Transition Analysis Model to Assess Change in Cognitive States over Three Occasions: Results from the Rush Memory and Aging Project. Journal Of Alzheimers Disease, 73(3), 1063–1073. https://doi.org/10.3233/JAD-190778
Examples of Research in Education
Schlatter, E., Molenaar, I., & Lazonder, A. W. (2021). Learning scientific reasoning: A latent transition analysis. Learning And Individual Differences, 92. https://doi.org/10.1016/j.lindif.2021.102043
Fryer, L. K. (2017). (Latent) transitions to learning at university: A latent profile transition analysis of first-year Japanese students. Higher Education, 73(3, Si), 519–537. https://doi.org/10.1007/s10734-016-0094-9
Reindl, M., Auer, T., & Gniewosz, B. (2022). Social Integration in Higher Education and Development of Intrinsic Motivation: A Latent Transition Analysis. Frontiers In Psychology, 13. https://doi.org/10.3389/fpsyg.2022.877072
Jeong, S., & Feldon, D. F. (2023). Changes in self-regulated learning profiles during an undergraduate peer-based intervention: A latent profile transition analysis. Learning And Instruction, 83. https://doi.org/10.1016/j.learninstruc.2022.101710
Gillet, N., Morin, A. J. S., & Reeve, J. (2017). Stability, change, and implications of students’ motivation profiles: A latent transition analysis. CONTEMPORARY EDUCATIONAL PSYCHOLOGY, 51, 222–239. https://doi.org/10.1016/j.cedpsych.2017.08.006
Brandes-Aitken, A., Braren, S., Swingler, M., Voegtline, K., & Blair, C. (2019). Sustained attention in infancy: A foundation for the development of multiple aspects of self-regulation for children in poverty. Journal of Experimental Child Psychology, 184, 192–209. https://doi.org/https://doi.org/10.1016/j.jecp.2019.04.006
About the author
Dr Oliver Perra is a lecturer at the School of Nursing and Midwifery, Queen’s University Belfast. His research revolves around the early experiences that explain differences in children’s adaptation and socio-cognitive abilities. He explores these issues by applying a transactional approach: this allows to investigate how interactions between children's characteristics and modifiable environmental factors can affect children's developmental pathways.
- Published on: 10 October 2023
- Event hosted by: Queen's University Belfast
- Keywords: Latent Transition Analysis |
- To cite this resource:
Oliver Perra. (2023). Introduction to Latent Transition Analysis. National Centre for Research Methods online learning resource. Available at https://www.ncrm.ac.uk/resources/online/all/?id=20821 [accessed: 22 November 2024]
⌃BACK TO TOP