Descriptive data and inferential statistics
Presenter(s): Chiara Dall’Ora
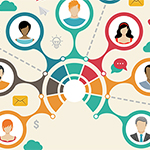
This tutorial covers the fundamentals of descriptive statistics and significance testing, two methods in data analysis. Descriptive statistics summarise data, providing the foundation for interpretation, while significance testing assesses whether observed differences are statistically meaningful. Together, they offer a comprehensive approach to analysing research data.
1. Descriptive Statistics
Descriptive statistics are numbers that help explain data in a table. They show simple details like average age, gender, or other characteristics of a group, which help understand the group or data before doing more complex analysis. For example, you might see descriptive statistics in a table showing how many people are male or female, or the average age of participants in a study.
It is important to describe data because data have a story to tell and, while it might be tempting to skip to the end of the book to find how the story ends, it often does not make much sense if we do not follow the whole plot. Without focusing on descriptive statistics first, it is difficult to spot trends and patterns, and to draw robust conclusions from your data.
This resource will explain how to read tables that contain descriptive statistics and significance testing.
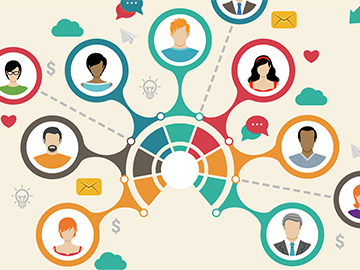
Tables reporting descriptive data analysis can be found in the results section of a paper, usually in the first table or Table 1. The first table usually summarises the study sample, so that readers understand how many participants made up the sample and what their characteristics were, for example, their demographic characteristics (i.e. age, sex, socioeconomic status, ethnicity). 1
When reading tables reporting descriptive statistics it is important to remind yourself of the research question. This will explain why the researchers decided to collect data on some variables and why they might have excluded other variables.
A descriptive table in a quantitative study might look like this (although there might be more variables or participant characteristics reported:
Table 1 Study outcome by participant characteristics of 8.073 participants:
Participant characteristics |
All (n = 8,073) | Experiencing outcome (n= 1,648) | Not experiencing outcome (n = 6,425) |
---|---|---|---|
Age. Mean (SD) | 70.8 (8.8) | 69.1 (9.2) | 71.3 (8.7) |
Gender. n (%) Female Male |
5,032 (62.3%) 3,041(37.7%) |
966 (58.6%) 682 (41.4%) |
3,948 (61.4%) 2,477 (38.6%) |
Highest level of qualification GSCE or equivalent A-levels or equivalent Undergraduate degree Postgraduate degree Doctorate |
625 (7.5%) 511 (6.4%) 3,927 (48.7%) 2,202 (27.3%) 808 (10.1%) |
84 (5.1%) 70 (4.3%) 880 (53.4%) 441 (26.8%) 173 (10.4%) |
541 (8.5%) 441(6.9%) 3,047 (47.4%) 1,761 (27.3%) 635 (9.9%) |
Examine the table in the following way:
Step 1:
Familiarise yourself with the sample – how many participants were included in the study? How many experienced the outcome in question (if you are reading an observational study) or how many were in the experimental and control groups?
Step 2:
Familiarise yourself with each variable or participant characteristic. These are usually reported by row, but this might vary by paper. Always check the table column and row names to be sure.
Step 3:
Note how researchers have decided to summarise each variable. It will depend on the type of variable, for example a numeric variable like age will often be reported as a mean and a standard deviation. Researchers might choose to categorise numeric variables and, for example, report age in age brackets, although categorising numeric variables is discouraged.2 Evaluate whether it is more valuable to know a variable’s mean/median/mode (measures of central tendency), their standard deviation or Interquartile Range (measures of dispersion) or whether it is better to display a variable as a categorical one.
Step 4:
Consider each variable and its distribution. Do you notice any major differences between groups, for example participants who experienced the outcome tending to be older than participants who did not experience the outcome? Or participants in the control group tending to be younger than in the experimental group?
Step 5:
Consider whether any difference that you notice in the different distributions might have implications for the overall study results. Always check your assumptions with the literature. For example, you might be worried that more people holding an undergraduate degree might influence their likelihood of experiencing the outcome – say being in employment, but is this correct? Do a quick literature search to find out.
Step 6:
If your assumption is correct, if you are reading an observational study, check how the authors have controlled for that variable in their analysis. If you are reading an experimental study, check the sampling and randomisation procedures. If they have been conducted correctly, it is safe to assume that any effect we observe is due to the intervention/experimental treatment and not due to differences in the variable distribution between intervention and control group. Researchers might have also used stratified sampling to reduce the imbalance between groups – which is a separate randomisation of subgroups of participants, for example, subgroups by age or gender.
2. Significance testing
Significance testing is a statistical method used to determine if observed differences between groups are meaningful or occurred by chance. This method is considered a controversial practice by many researchers across several disciplines,3-6 with numerous instances of such tests being conducted and reported incorrectly.
In a nutshell, significance testing aims to draw conclusions about a population based on data drawn from a sample. It requires researchers to formulate a null hypothesis about the population, meaning that there are no differences between groups in the population. Significance tests will either confirm or reject the null hypothesis – indicating that there are differences in the population. The problematic aspect of these tests is that they are often used to demonstrate that there was an effect of, for example, the experimental treatment on an outcome. Nonetheless, such tests cannot achieve this level of sophistication.
Typical tests include the t-test, the Mann-Whitney and chi-square test. Before considering these tests, it is worth mentioning the p-value – which is the frequency probability of the distribution meeting the null hypothesis. It is the standard metric researchers use to reject or fail to reject the null hypothesis, and it is typically set at <.05.
When reading a table reporting a t-test, this is what you would normally see:
T-value | p-value |
4.1729 | .0001 |
The t-value itself does not convey much meaning – there are tables you can consult to look at the different t-values and their corresponding level of statistical significance but let us focus on the p-value. The p-value is the frequency probability of the distribution meeting the null hypothesis. Let us assume that in this case, the null hypothesis is that the mean ages of the two populations are equal. This becomes problematic because we do not have two populations. We have a sample, drawn from a single population, so this t-test is not particularly helpful. The p-value is .0001. This means that the frequency probability of meeting the null hypothesis is incredibly low, so we can reject it. We should not attach any meaning to this because the assumptions of this test (checking if two population means are equal) are not valid in a study drawing on a sample from a single population.
The chi-square test is appropriate for categorical variables. It measures the discrepancy between the observed and expected counts where the null hypothesis fails to be rejected (in this case the null hypothesis is that gender distribution is equal between the two populations). This is what researchers will typically report:
Χ2 | p-value |
0.9726 | .324 |
Similarly to the above, you should focus on the p-value. It is .324, so it’s higher than .05, which is the standard threshold for statistical significance. This means that we cannot reject the null hypothesis.
As a final remark, these tests tend to be controversial and often wrongly applied, but they are still reported in many papers, so you need to know how to interpret them when you find them in papers, but with the caveat of always checking the underlying assumptions of such tests.
> Download an exercise worksheet (with answers)
For practical tips to aid data visualisation, there is an excellent resource.
About the author
Chiara qualified as a Registered Nurse in 2011. In 2018, she was awarded a PhD in Health Sciences at the University of Southampton. Since 2023, Chiara has been an Associate Professor within the Health Workforce & Systems theme in the School of Health Sciences. Chiara leads a research programme to improve health workforce wellbeing and performance, with a specific focus on work hours and workforce configuration and patient safety. She draws on large workforce and patient datasets and applies multilevel modelling techniques.
- Published on: 18 October 2024
- Event hosted by: Southampton
- Keywords: Descriptive statistics | inferential statistics | significance testing | t-test | p-value | chi-square test Descriptive Statistics | Statistical Theory and Methods of Inference | Experimental Research |
- To cite this resource:
Chiara Dall’Ora. (2024). Descriptive data and inferential statistics. National Centre for Research Methods online learning resource. Available at https://www.ncrm.ac.uk/resources/online/all/?id=20846 [accessed: 21 November 2024]
⌃BACK TO TOP