Multilevel models to study intersectionality
Presenter(s): Andrew Bell and George Leckie
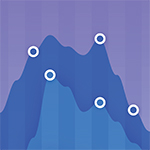
Multilevel models allow researchers to analyse data that has a clustered structure such as pupils nested within schools, or individuals within neighbourhoods. Recently, a version of multilevel models has been developed for the study of intersectional inequalities in individual outcomes – the Multilevel Analysis of Individual Heterogeneity and Discriminatory Accuracy (MAIHDA). With MAIHDA, individuals are modelled as nested within their intersectional strata, which are the combinations of various sociodemographic identity categories observed in the data, for instance, gender, age, ethnicity and social class. This quantitative method allows researchers to assess both individual and intersection-level variation in individual outcomes.
This resource explains the implementation of MAIHDA including through statistical software R or Stata, providing step-by-step videos and worksheets.
What is intersectionality?
An intersectional approach recognises that individuals are shaped by multiple social identities, and these identities intersect, through social processes of social power and discrimination. This produces complex inequalities, as multiple processes combine to produce particular ways to advantage and disadvantage particular groups of people with particular combinations of identities.
Traditional regression models may struggle to capture the full complexity of these interactions because they often treat identities as separate, independent variables. MAIHDA, however, accounts for the fact that these identities do not exist in isolation but interact in ways that amplify or mitigate inequalities. This approach makes it possible to move beyond additive models and explore how intersectional identities create compounded effects.
How Does MAIHDA Work?
MAIHDA consists of two models:
- A null model (random intercepts model): This model includes only the group-level (strata) random effects, allowing researchers to estimate the variation across different intersectional groups without accounting for any specific individual characteristics.
- A main effects model: This model adds individual characteristics (such as race, gender, income, etc.) as fixed effect explanatory variables. This allows us to estimate the extent to which the inequalities identified in the null model are produced by universal, additive effects of the social identities used to define the intersections, and the extent to which they are produced by multiplicative combinations of those variables
We can use these models to identify two key statistics. First, the Variance Partitioning Coefficient (VPC) in model 1 will tell us the extent to which there is variation between strata, and is calculated as the strata level variance divided by the total variance. This statistic measures the overall importance or size of the intersectional inequalities. Second, The Proportional Change in Variance (PCV) tells us how much of the strata variance in the null model is explained by the additive effects of the social identities, with the remainder being multiplicative, that is due to hidden and complex combinations of those identities. The PCV is calculated as the change in strata-level variance between model 1 and 2, divided by the null model’s strata-level variance.
Challenges and Considerations
There are a number of challenges that MAIHDA users should consider when using and interpreting the MAIHDA method:
Sample Size: MAIHDA requires a sufficiently large sample size, especially when analyzing rare or small intersectional groups. If the strata sizes are too small, the predicted stratum means will be shrunk back towards what would be expected given their additive effects, meaning any multiplicative effects won’t be fully estimated. Researchers therefore need to balance the need for complexity in the model with the limitations of the sample size. This choice will depend on the specifics of the research question, and the nature of the theory underlying the research question at hand.
Interpretation of Results: Understanding and interpreting the multiplicative effects in MAIHDA requires a solid grasp of both the statistical and theoretical foundations of intersectionality. MAIHDA can produce a large range of results, and telling a story through those results is often challenging and at risk of bias
How intersectional is MAIHDA? MAIHDA is a robust method for estimating inequalities in means between subgroups of a population. It doesn’t tell us anything about the processes underlying those inequalities, and so is not, in itself, intersectional. Intersectionality comes into the variables that are chosen to define the strata, and the interpretation and theoretical lens through which those inequalities are analysed. MAIHDA can be used in a non-intersectional way, using non-identity variables in ways that don’t particularly relate to social power structures.
How to report and visualise results from MAIHDA
Researchers should report the estimates of the two models next to each other, so readers can compare, in particular the strata-level variance. It is generally sensible to report the VPC of both models, and the PCV.
Plots are often used to report the predicted stratum means, and there are a number of different choices that a researcher can make. They can be plotted as a single “caterpillar plot”, either ordered by rank or by particular strata-defining variables. The choices made in these plots (for instance in the ordering of variables) may influence the story of the results that is told, and so should be thought about carefully.
Researchers may also want to plot the predicted interaction effects (that is, the predicted random effect values from model 2). However, these should be interpreted carefully. They do not directly predict advantage or disadvantage – rather they predict a difference in (dis)advantage compared to what might the expected given the additive effects. They are useful for identifying those strata whose predicted means differ from what we would naively expect base only on the additive main effects.
Extensions to MAIHDA
MAIHDA can be extended in a variety of ways, just like any multilevel model:
- Control for variables (that don’t define the strata) to consider how variability controlling for other things is patterned
- Add two-way interactions as fixed-effect explanatory variables to help understand the nature of the multiplicative variability
- Add random slopes to see how the effect of a particular variable (for instance a policy intervention) varies across strata
- Add levels, for instance to incorporate geography
Practical exercises
Below are some practical exercises that you can work through, along with explainer videos, in both Stata and R.
> Download a ZIP folder with files: Practical R, Practical Stata, R code, Statacode, and TutorialData.
This tutorial came about in part from work conducted as part of a Economic and Social Research Council grant, number ES/X011313/1.
About the author
Dr Andrew Bell is a Senior Lecturer in Quantitative Social Sciences, Sheffield Methods Institute, University of Sheffield. Methodologically, Andy’s interests are in the development and application of multilevel models, with work focusing on methods for uncovering intersectional inequalities, age-period-cohort analysis and fixed and random effects models. His substantive research spans a range of disciplines, including geography, political science, social epidemiology and economics.
https://www.sheffield.ac.uk/education/people/academic/smi/andrew-bell
Prof. George Leckie is a Professor of Social Statistics and Co-Director of the Centre for Multilevel Modelling (CMM) at the University of Bristol, UK. His research interests focus on the application and dissemination of multilevel modelling. He has applied this method to study the effects of institutional and geographical contexts on sociodemographic inequalities in individual educational and health outcomes. A long-standing interest of his has been examining school effects on student learning through school value-added models, as well as engaging in debates regarding the use of such data to inform school accountability and choice.
https://www.bristol.ac.uk/cmm/team/leckie.html
- Published on: 30 January 2025
- Event hosted by: Sheffield/Bristol
- Keywords: multilevel models | intersectionality | MAIHDA | individual outcomes | statistical analysis |
- To cite this resource:
Andrew Bell and George Leckie. (2025). Multilevel models to study intersectionality. National Centre for Research Methods online learning resource. Available at https://www.ncrm.ac.uk/resources/online/all/?id=20849 [accessed: 17 April 2025]
⌃BACK TO TOP