Synthetic Control Methods - Introduction
Presenter(s): Xingna Zhang
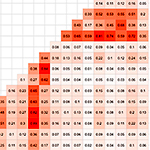
The synthetic control method is a robust statistical approach used to estimate causal effects in situations where randomised controlled trials (RCTs) are impractical or impossible to conduct. It is particularly valuable for evaluating the impact of interventions — such as policy changes, health programmes, or other treatments — on specific outcomes using observational data. The method is widely applied in fields like economics, public health, and policy evaluation, where RCTs may be unethical, costly, or simply unfeasible.
The method works by constructing a "synthetic control group" that closely resembles the treated unit (the group, region, or entity that received the intervention) in the pre-intervention period. This synthetic group is created by combining and optimally weighting multiple untreated control units, ensuring that it matches the treated unit’s characteristics and behaviour before the intervention. The synthetic control group then serves as a counterfactual — a representation of what would have likely happened to the treated unit if the intervention had not occurred. By comparing the post-intervention outcomes of the treated unit to those of the synthetic control group, researchers can estimate the causal effect of the intervention.
Strengths
The method provides a transparent and data-driven way to estimate causal effects in the absence of RCTs. Unlike traditional regression-based approaches, which often rely on strong assumptions about functional forms, the synthetic control method uses a weighted combination of control units to construct a more credible counterfactual. This makes it particularly useful for case studies involving a single treated unit, such as evaluating the impact of a policy change in a specific country or region.
Additionally, the method is flexible and can be adapted to a wide range of settings, from assessing the economic costs of the terrorist violence, to evaluating the effectiveness of a public health programme. Its reliance on pre-intervention data to construct the synthetic control group also helps reduce concerns about post-intervention confounding, as the weights are determined based on historical trends rather than outcomes influenced by the intervention.
Limitations
One major challenge is the requirement of a sufficiently long pre-intervention period to ensure that the synthetic control group accurately reflects the treated unit’s characteristics. If the pre-intervention period is too short, the synthetic control may not provide a reliable counterfactual, leading to biased estimates of the intervention’s effect.
Another limitation is the method’s reliance on the availability of comparable control units. If there are no suitable control units that can be combined to closely match the treated unit, the synthetic control group may not adequately represent the counterfactual scenario. This can undermine the validity of the causal inference.
Finally, the synthetic control method assumes that the relationship between the treated unit and the control units remains stable over time. If unobserved factors influence the treated unit differently after the intervention, this assumption may be violated, potentially leading to inaccurate conclusions.
Practical considerations
There are several practical considerations to keep in mind when applying it, to ensure that the method is used effectively and that the results are reliable and meaningful.
1. Data Quality and Availability
The success of the synthetic control method depends heavily on the quality and availability of data. You need detailed data on both the treated unit (the group or region that received the intervention) and potential control units (those that did not receive the intervention). This data should cover a sufficiently long pre-intervention period to accurately capture trends and characteristics. If the data is incomplete, inconsistent, or only available for a short time frame, the synthetic control group may not be a reliable counterfactual, leading to biased results.
2. Choosing Control Units
Selecting appropriate control units is critical, which should be similar to the treated unit in terms of characteristics and pre-intervention trends. For example, if you’re evaluating a policy change in one region, you’d want to compare it to regions with similar economic, social, and political conditions. If the control units are too different, the synthetic control group won’t accurately represent what would have happened to the treated unit without the intervention. This can weaken the validity of your findings.
3. Pre-Intervention Fit
The synthetic control group should closely match the treated unit in the pre-intervention period. This means that the weighted combination of control units should replicate the treated unit’s outcomes and characteristics as closely as possible. If the fit is poor, the synthetic control method may not provide a credible counterfactual. There are established statistical measures to assess how well the synthetic control matches the treated unit before the intervention.
4. Handling Missing Data
If key variables or time periods are missing for some control units, it may be difficult to construct a reliable synthetic control group. Researchers often need to decide whether to exclude units with missing data or use imputation techniques to fill in the gaps. However, imputation can introduce uncertainty, so it’s important to carefully consider the trade-offs.
5. Sensitivity Analysis
You need to test the robustness of your results, including checking how sensitive your findings are to changes in the control units, weights, or time periods. For example, you might exclude certain control units or vary the pre-intervention period to see if the results hold. If the findings are highly sensitive to these changes, it may indicate that the synthetic control group isn’t a reliable counterfactual.
6. Interpreting Results
You need to interpret the results cautiously. Unobserved factors or changes that occur after the intervention could still influence the results. It’s important to clearly communicate the limitations of the analysis and avoid overstating the conclusions.
7. Software and Implementation
Implementing the synthetic control method requires specialised statistical software, such as R, Stata, or Python. These tools often have specific packages or libraries designed, but using them effectively requires some technical expertise. If you’re not familiar with the software, you may need to collaborate with someone who has the necessary skills.
> Video 2: download R file used in video 2.
> Worksheet: download exercise (example data csv, instruction word document, and R file).
> Download a step by step process.
About the author
Xingna Zhang is a Tenure Track Fellow at University of Liverpool. She is a geographer and studies health inequalities. Her recent work focuses on using natural experiments to evaluate the impact of policies upon health inequalities –– a niche area in the intersection of Human Geography and Public Health. She currently leads on researching autism diagnosis impact on health and education outcomes, funded by Administrative Data Research UK (ES/Z502431/1).
- Published on: 3 April 2025
- Event hosted by: University of Liverpool
- Keywords: Synthetic Control | Natural experiments | Case studies | Causal inference | Quasi-experiments | Evaluation research | Statistics |
- To cite this resource:
Xingna Zhang. (2025). Synthetic Control Methods - Introduction. National Centre for Research Methods online learning resource. Available at https://www.ncrm.ac.uk/resources/online/all/?id=20854 [accessed: 17 April 2025]
⌃BACK TO TOP